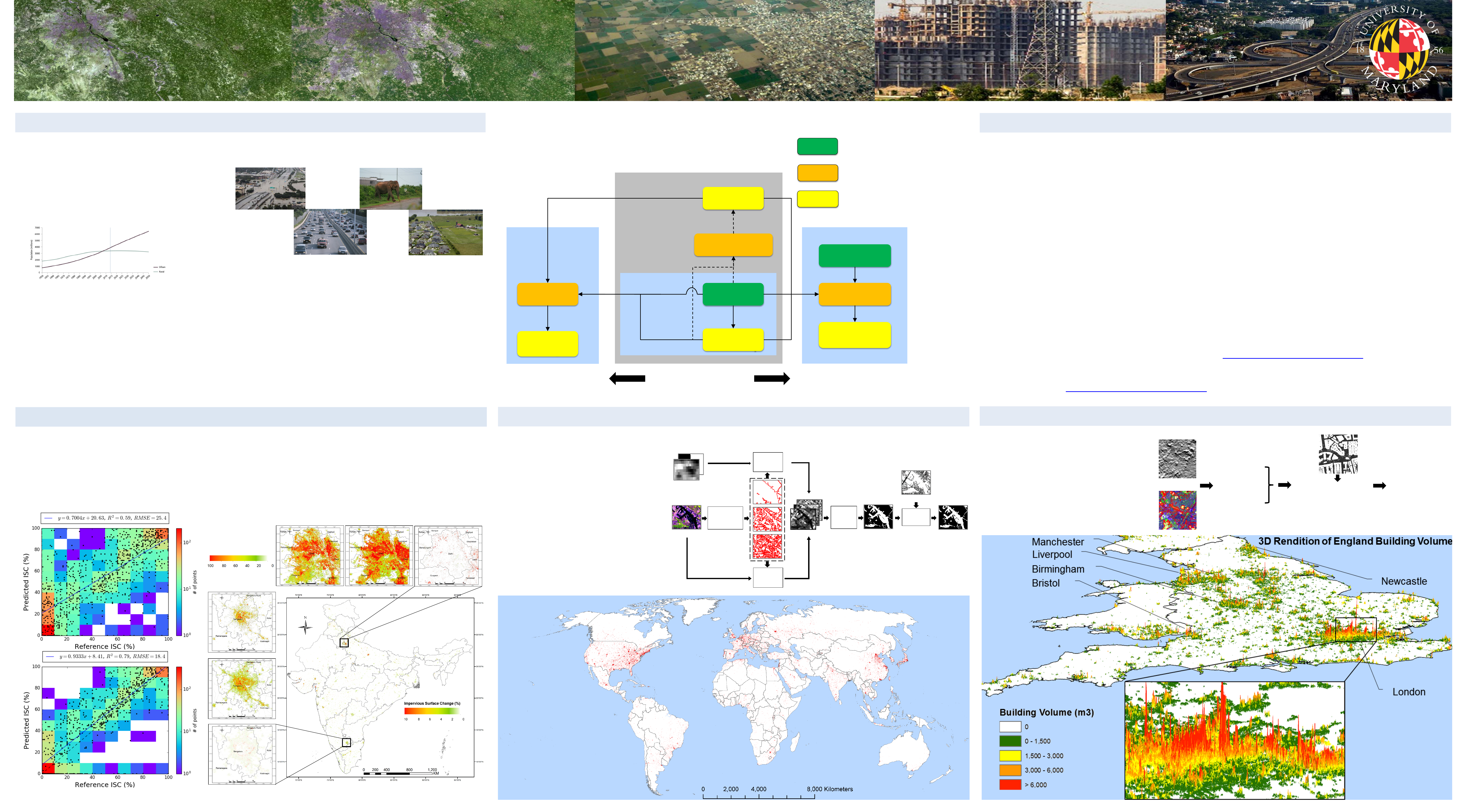
3D Urban Structure
Background
Urban Extent
Conclusion
Sub-pixel Urban Change
Towards Fine Scale Characterization of Global Urban Extent, Change and Structure
Panshi Wang
Department of Geographical Sciences, University of Maryland
Landsat
Data
Impervious
Surface
Urban
Extent Map
GMIS Project
ITP
Sub-pixel
Change
GMIS Mapping
Method
Global DSM
Height metrics
Building
height/volume
Urban Extent
Urban Change
Urban 3D Structure
2D Urban FormTemporal Domain Vertical Domain
Input Data
Method/
Intermediate results
Output/Final Product
*
GMIS stands for Global Man-made Impervious Surface.
*
IS2000
IS2010
ISC
IS2000 IS2010 ISC
New Delhi
Bengaluru
Zoom-in View Impervious Surface (%)
2000 – 2010 Impervious Surface Change (ISC) of India
Landsat image
Feature set
VIIRS-DNB &
MODIS-NDVI
Non-texture
features
calculation
Texture
features
calculation
Random
Forest
Segmentation
&
level selection
Post-
processing
OpenStreetMap
Research Objectives: better quantification of global urban extent,
urban change, and 3D urban structure.
Global circa-2010 map of Human Built-up And Settlements Extent (HBASE)
• Effective methods were developed for the quantification of urban extent, sub-pixel urban change and 3D urban
structure.
• The proposed urban extent mapping methodology has demonstrated global applicability and may be used for
fully automated update of global urban extent maps.
• Using the iterative algorithm, sub-pixel impervious surface change could be mapped accurately with spectrally
inconsistent bi-temporal imagery.
• Freely available global elevation datasets have a potential for mapping building volume, a much needed
information for population, sustainable urban design and disaster vulnerability applications.
Acknowledgements:
• This study was partially funded by NASA’s Land Use and Land Cover Change (LULCC) program (NNX11AH67G).
• Global Land Cover Facility (GLCF), University of Maryland
References:
• Wang, P.; Huang, C.; Brown de Colstoun, E.C. Mapping 2000–2010 Impervious Surface Change in India Using
Global Land Survey Landsat Data. Remote Sens. 2017, 9, 366.
• Brown de Colstoun, E. C., C. Huang, P. Wang, J. C. Tilton, B. Tan, J. Phillips, S. Niemczura, P.-Y. Ling, and R.
E. Wolfe. 2017. Global Man-made Impervious Surface (GMIS) Dataset From Landsat. Palisades, NY: NASA
Socioeconomic Data and Applications Center (SEDAC).
https://doi.org/10.7927/H4P55KKF
• Wang, P., C. Huang, E. C. Brown de Colstoun, J. C. Tilton, and B. Tan. 2017. Global Human Built-up And
Settlement Extent (HBASE) Dataset From Landsat. Palisades, NY: NASA Socioeconomic Data and Applications
Center (SEDAC).
https://doi.org/10.7927/H4DN434S
+
DSM
Landsat-based
Segmentation
Height Metrics
Random Forest Regression
Lidar-based Height/Volume
Ancillary Data
(e.g., impervious
surface)
Building Height/Volume
Urbanization presents challenges to humanity in terms of :
• Local-to-regional climate and hydrology
• Energy consumption and emissions
• Loss of agricultural land and food security
• Biodiversity
• Structural socioeconomic change
Good remote sensing data on urban extent, change, and structure are essential for:
• Consistent definition of “urban” for large-scale urban studies
• Long-term monitoring/modelling of the trend of urbanization
• Linking changes in urban form and structure with socioeconomic conditions
• Determining the sustainability of urban growth
• Assessing the vulnerability of urban areas to natural hazards
• Policy making based on scientific evidence
Scatterplots between predicted and reference impervious
surface change (ISC) : (a) before ITP, (b) after ITP
(a)
(b)
Urban extent mapping method using multi-level object-based texture and non-texture features
Challenges of global urban
extent mapping:
• Only Global Land Survey
available at the time of the study
• Differences in acquisition
date/phenology
• Spectral confusions and
inconsistencies
• Ambiguities in definition
• Height information from global
digital surface models (DSMs)
• Landsat-derived objects used for
height information extraction
• A suite of object-based height
metrics derived for machine
learning regression algorithm
• Multiple DSM datasets fused
• Sub-pixel mapping necessary for characterizing urban change at 30m resolution
• Built on a mature regression tree (RT) based impervious surface algorithm used by the GMIS project
• Training data only available for one date: 2010
• Training data for 2000 automatically generated using an Iterative Training and Prediction (ITP) algorithm